讲座主题:Optimal embedding for reversible data hiding: Theoretical investigation and practical algorithm
主讲专家:李晓龙
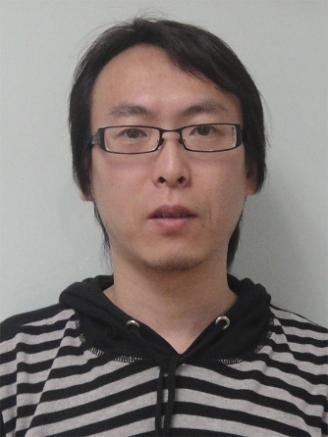
主 持 人:周晓谊(副经理)
报告时间:2023年04月07日15:30-17:00
报告地点:线上会议号:669 153 900(腾讯会议)
专家简介:Prof. Xiaolong Li received the B.S. degree from Peking University (China), the M.S. degree from Ecole Polytechnique (France), and the Ph.D. degree in mathematics from ENS de Cachan (France), in 1999, 2002, and 2006, respectively. He worked as a postdoctoral fellow and then a researcher at Peking University in 2007–2016. He is currently a Professor with the Institute of Information Science, Beijing Jiaotong University. His research interests are image processing and information hiding. Prof. Li has published more than 40 SCI journals (including 15 IEEE transactions papers) and 50 international conference papers. According to Google citation, Prof. Li’s academic results have been cited more than 3900 times with h-index 27.
报告简介:Reversible data hiding (RDH) is a specific data hiding technique in which both the embedded data and the original cover image can be exactly extracted from the marked image. In this paper, we present a general expansion-shifting model for RDH by introducing the so-called reversible embedding function (REF) which maps each point of Z^n to a non-empty subset of Z^n. Moreover, to guarantee the reversibility, the mapped subsets of distinct points should be disjointed to each other. With REF, RDH can be designed and the corresponding rate-distortion formulations can be established, providing an approach to optimize the embedding performance. Some examples of REF are given for a better illustration, and a preliminary theoretical investigation on REF is conducted as well. Then, optimal REF for one-dimensional histogram is investigated, and all optimal REF are derived in this case when the maximum modification to the cover pixel is limited as a small value. Finally, based on the optimal REF for one-dimensional histogram and the idea of multiple histograms modification, a practical RDH scheme is presented and it is experimental verified better than some state-of-the-art algorithms in terms of capacity-distortion performance.